The whole is equivalent to the sum of its parts
Which is bigger, 10 or 7?
I suspect that for most, the response to this question is instinctive, unconscious, and immediate. So how about I pose a follow-up question:
How do you know?
If you can refrain from dismissing this question as trivial, I invite you to pause and try to reflect on what happened in your mind in that instant – is this factual recall, was there something visual, was it something contextual, or was it something else?
Perhaps you will indulge me and delve a little deeper:
In how many ways do you know?
Here again, I invite you to pause and consider your response before continuing. Maybe you would like to imagine that you are trying to convince someone or different people. Pick up a piece of paper and draw pictures, write things down, and try to form another approach that is different in some way from the others.
When we compare numerical values, there are many helpful approaches that we can take. These might be based on processes such as: counting, “1, 2, 3, 4, 5, 6, 7, 8, 9, 10. I said 7 first so it must be smaller (that’s how numbers work!)”; motion/movement, “If we start together at the bottom, then I climb 7 stairs and you climb 10 stairs, you will be higher than me (and more tired!)”; measurement/length, “This length (7cm) is shorter than this length (10cm)”; matching/creating correspondences, “There are 10 people and 7 cupcakes if I hand out a cupcake to each person I will run out – not everyone will get one!”
Each of these approaches (and the many more you might imagine) might be grounded in two prominent types of reasoning: part-whole and/or correspondence. These two ideas are used pervasively, interchangeably, and often simultaneously when reasoning with numbers in most of school mathematics and in our daily experiences.
Part-whole
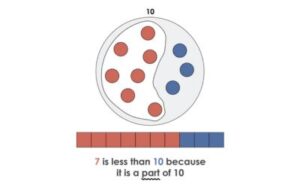
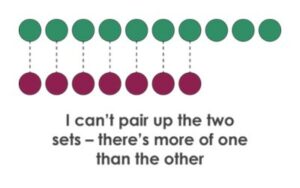
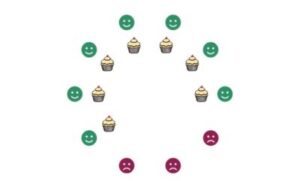
Let’s take another comparison problem, this time inspired by questions posed to children in a study by Falk (2010):
What are there more of:
- Hairs on your head OR fingers on two hands?
- Fingers on two hands OR days in a month?
- Grains of sand on Earth OR hairs on your head?
- All numbers OR grains of sand on Earth?
This time, I suspect, your responses were not always instantaneous and more conscious thought was required. How convinced are you of your responses? Did you feel as though more information was required?
When you reflect on the reasoning you employed in making these comparisons, I wonder whether you assigned numerical values to the quantities – did you feel an urge to do so, as a first step, before applying similar techniques to those used before?
When the children in this study were faced with such comparisons, an interesting misconception revealed itself: many of them considered a very large number, for instance, the number of grains of sand on Earth, to be synonymous with infinity. This, of course, presents a potential difficulty with question 4; I suspect you won’t be alone if you encounter this, too.
When we encounter numbers or quantities that are so large/vast that they are beyond our comprehension, it is perhaps unsurprising that we equate these with infinity – that magical word that creeps into our consciousness from a very young age as the default answer to any questions about “biggest number.” So, is this a problematic concept to hold? In practical terms, for most people, probably not. But mathematically it is, and actually confronting it offers some wonderful opportunities to explore, discuss and better understand the numbers that we work with, the structure of mathematical systems, and the nature of the mathematics that we study.
So how could we confront this misconception? How can we take advantage of the opportunities alluded to above? Well, one possibility is purposefully to create situations where the misconception might arise.
Position the quantities representing the grains of sand on Earth and all numbers on a number line.
- Would they be in the same place, or would one be closer to zero than the other?
- If they are not in the same place, are they very close together or very far apart?
- Is it possible to measure the gap between these two quantities?
Talking around this task is likely to draw attention to the fact that some quantities may be large and unknown, but we can be certain they are finite – a single number exists to represent them, we just don’t know what it is. Others, however, are large, unknown, and also not finite – they are not represented by a single large number but are unbounded, often the result of an infinite process such as counting. These infinite quantities cannot be positioned on a number line, and the gap (the difference) between any finite quantity and an infinite one is immeasurable – it is infinitely large in itself!
So, is it possible to make comparisons with infinite quantities? Or is this “not allowed?!” Well, we can certainly say that any finite quantity is smaller than any infinite quantity. But how about this:
What are there more of: natural numbers or even numbers?
I would encourage you, once again, to establish and hold your own response to this question in your mind before reading on.
As at the beginning of this blog, the follow-up question is:
How do you know?
Intuition tends to be strong here, grounded in our experiences with finite quantities and part-whole reasoning: the even numbers are a part of the natural numbers so there must be more natural numbers (twice as many, we might argue). We can confirm this with examples; for instance, by comparing the number of natural numbers and even numbers there are up to a fixed point, say 100:
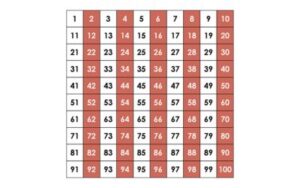
Now, what if I asked you to find an alternative approach, another way of explaining how you know that there are more natural numbers than even numbers? When we compared 7 and 10, we discussed two main approaches, those based on part-whole reasoning and those based on matching / correspondences. What would a correspondence approach look like here?
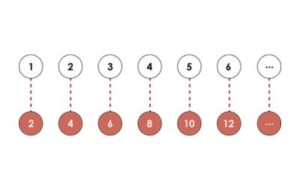
It looks as though I can pair up the two sets of numbers, I can match every natural number, one-to-one, with an even number, so the two sets are equal… Uh oh! And, more than that, our two methods of comparison, which are usually used interchangeably, lead to different results!
How do you feel about this seemingly contradictory situation? Maybe this example is something you are comfortable with, but most likely not! For many students, and indeed teachers, this is a troubling situation, causing us to throw up our hands in despair and confusion! However, if we can overcome this sensation and recognise that the conflict is real (it’s not that we’ve made an error), then the stage is set for thinking more carefully about assumptions that might have been made and when and where our mathematical rules and procedures are used and valid. Giving students similar opportunities to encounter situations where their intuition is called into question, inviting them to discuss (and argue!), expose their own lines of reasoning, and compare contexts and situations in the search for an explanation, is surely a good thing! Perhaps, when prompted in this way they might also be more receptive to the introduction of standard, accepted approaches within mathematics.
As a closing comment, let’s notice that our discussions are touching on the most fundamental property of any infinite set: that it can be matched, one-to-one, with a proper subset of itself. In other words, in the case of infinite sets, the whole is equivalent to some of its parts!
For more insights like this, visit our website at www.international-maths-challenge.com.
Credit for the article given to Tabitha Gould